Ever wondered how foundation agents can revolutionize AI decision-making in the real world? Imagine a future where complex choices are streamlined, outcomes optimized, and efficiency maximized. Foundation agents hold the key to unlocking this potential, reshaping industries and enhancing user experiences. What if your decisions were not just data-driven but empowered by advanced algorithms that learn and adapt in real-time? Dive into the realm of AI decision-making with foundation agents at the forefront, paving the way for smarter, faster, and more accurate choices. Join us as we explore the transformative impact of these agents on decision-making processes across various sectors.
Table of Contents
AI Decision-Making Challenges
Real-world complexities
Foundation agents face significant challenges when applied in complex real-world environments. Adapting to dynamic and unpredictable scenarios poses a major hurdle for these AI systems. The intricacies of decision-making processes in such settings require quick and accurate responses.
In real-world applications, foundation agents must navigate uncertainties and diverse factors. Their ability to adapt to changing conditions is crucial for effective decision-making. Flexibility and resilience are key traits that enable these AI systems to thrive in varied environments.
Navigating through the complexities of real-world scenarios demands robust algorithms and continuous learning capabilities. Foundation agents must be equipped with mechanisms to handle unexpected events and evolving data inputs. Dynamic decision-making processes are essential for their success.
Data limitations
Data scarcity significantly impacts the training of foundation agents. Limited datasets can hinder the AI system’s ability to generalize well across different scenarios. Ensuring sufficient and diverse data is crucial for enhancing decision-making capabilities.
Challenges related to data quality and diversity further complicate the training process of foundation agents. Biased or incomplete datasets can lead to skewed outcomes and inaccurate predictions. Maintaining high-quality data sources is essential for optimal performance.
To overcome data limitations, strategies such as data augmentation and transfer learning can be employed. These techniques help enhance the diversity and richness of the dataset used for training foundation agents. Continual monitoring and evaluation of data quality are also vital for sustained performance.
Bias and fairness
Decision-making processes using foundation agents may inadvertently introduce biases into outcomes. Understanding the potential biases that can arise is critical for ensuring fair and equitable results. Detecting and addressing bias is essential for maintaining trust in AI systems.
Ensuring fairness in AI outcomes is paramount for upholding ethical standards in decision-making tasks. Striving for transparency and accountability in the deployment of foundation agents helps mitigate biases effectively. Fairness-aware algorithms play a crucial role in promoting equitable decisions.
Mitigating bias requires implementing bias detection mechanisms and incorporating fairness considerations into the model development process. Regular audits and reviews can help identify biased patterns early on, allowing for timely interventions to rectify any unfair practices.
Ethical considerations
Utilizing foundation agents for decision-making tasks raises various ethical concerns that need careful consideration. Developers bear the responsibility of designing AI systems that align with ethical standards, prioritizing values such as transparency, accountability, and privacy.
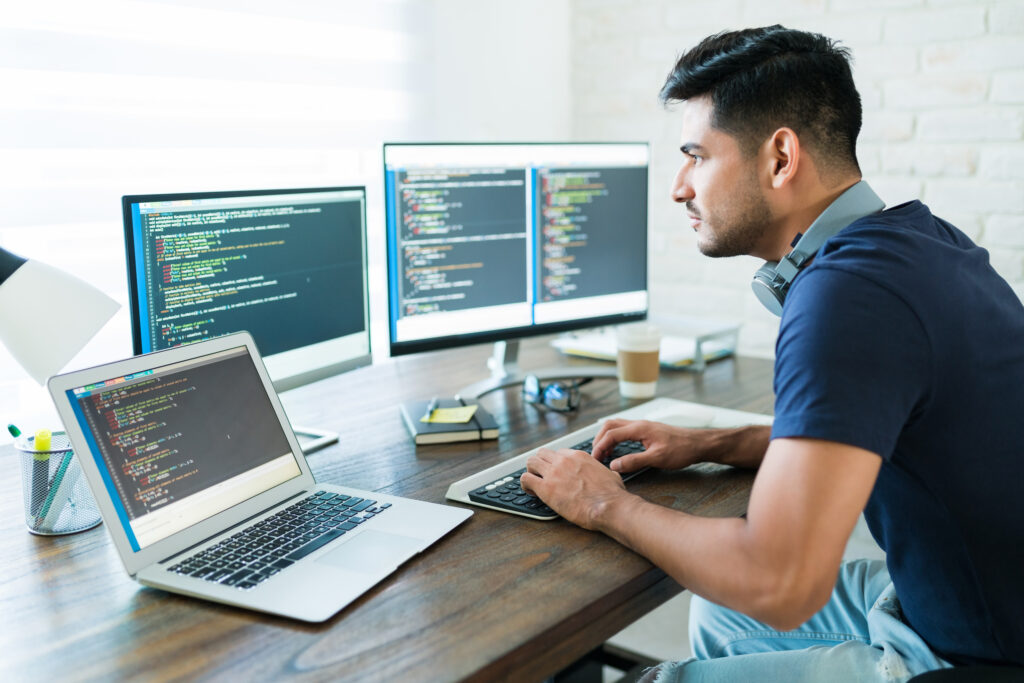
Ethical frameworks provide guidelines for integrating foundation agents into real-world applications responsibly. Upholding principles of beneficence, non-maleficence, autonomy, and justice ensures that AI technologies contribute positively to society while minimizing harm.
Integrating ethical considerations into every stage of AI development—from design to deployment—is essential for fostering trust among users and stakeholders. Adhering to ethical norms not only safeguards against potential risks but also paves the way for sustainable innovation in AI technologies.
Understanding Foundation Agents
Key Characteristics
Foundation agents stand out from traditional AI systems due to their unified representations and advanced reasoning capabilities. Unlike conventional AI, foundation agents can integrate data from various sources into a cohesive knowledge base for decision-making. This unique trait allows them to process information more effectively.
The significance of unified representations lies in the ability of foundation agents to synthesize diverse data types into a single coherent framework. By unifying information across different modalities, such as text, images, and videos, these agents can make more informed decisions based on a holistic understanding of the input.
Multi-modality perception and cross-domain adaptation are key features that empower foundation agents to excel in decision-making tasks. The ability to perceive and interpret information from multiple sources enhances their cognitive capabilities, while cross-domain adaptation enables them to apply learned knowledge across different contexts.
Enhancing Decision Processes
Foundation agents revolutionize decision processes by leveraging advanced reasoning mechanisms and comprehensive world knowledge. Through sophisticated reasoning algorithms, these agents can analyze complex scenarios and generate optimal solutions based on logical deductions.
By incorporating feedback signals and real-time environment states, foundation agents continuously refine their decision-making outcomes. This iterative process allows them to adapt to changing circumstances and improve the accuracy of their decisions over time.
The efficiency of foundation agents shines through in their adeptness at handling intricate decision tasks across diverse domains. Whether it’s financial forecasting, medical diagnosis, or autonomous driving, these agents demonstrate remarkable versatility in navigating complex decision landscapes.
Overcoming Challenges
Developing and deploying foundation agents in real-world settings comes with its share of challenges. One major obstacle is the need to address data discrepancies and ensure seamless integration of information from disparate sources. Bridging domain gaps is crucial for enhancing the overall performance of foundation agents.
To tackle challenges related to data quality and domain specificity, strategies focusing on robust data preprocessing and feature engineering are essential. By refining the input data and optimizing feature extraction processes, developers can enhance the learning capabilities of foundation agents.
Innovative solutions play a vital role in overcoming technical hurdles associated with implementing foundation agents. From leveraging transfer learning techniques to developing adaptive algorithms for dynamic environments, continuous innovation is key to maximizing the potential of these advanced AI systems.
Revolutionizing Decision Making
Addressing Real-World Issues
Foundation agents have brought a paradigm shift in decision-making by offering unique approaches to solving problems. These agents analyze vast amounts of data to generate insights that guide strategic choices. By incorporating diverse perspectives, they enhance decision quality and accuracy. In various industries, from healthcare to finance, foundation agents play a crucial role in optimizing processes and outcomes.
The potential impact of foundation agents on industries is immense. Their ability to process complex information swiftly allows for quicker and more informed decisions. In healthcare, for example, these agents assist medical professionals in diagnosing illnesses accurately and recommending effective treatments. Moreover, in addressing societal challenges such as climate change or poverty, foundation agents provide valuable insights that inform policy decisions and resource allocation strategies.
Case studies exemplify the successful application of foundation agents in resolving real-world issues. For instance, in the field of autonomous vehicles, these agents enable cars to make split-second decisions based on real-time data analysis. This technology has significantly improved road safety and efficiency by reducing human errors. Similarly, in customer service, foundation agents streamline interactions through personalized recommendations and efficient problem-solving techniques.
Key Features for Success
The success of foundation agents hinges on their essential features and capabilities that set them apart from traditional decision-making systems. Adaptability is a key trait that allows these agents to learn from new data inputs and adjust their strategies accordingly. This flexibility ensures that they can handle evolving scenarios effectively and make informed decisions in dynamic environments.
Another critical aspect is generalization, which enables foundation agents to apply knowledge gained from one domain to another. This capability enhances their problem-solving skills by drawing parallels between different situations and extracting relevant insights. By generalizing concepts across various contexts, these agents demonstrate versatility and efficiency in decision-making processes.
Furthermore, policy interfaces play a vital role in optimizing the performance of foundation agents. These interfaces define the rules and guidelines that govern agent behavior, ensuring ethical conduct and alignment with organizational objectives. By integrating policy frameworks into their decision-making algorithms, foundation agents uphold integrity and transparency while delivering consistent results across diverse tasks.
Roadmap for Implementation
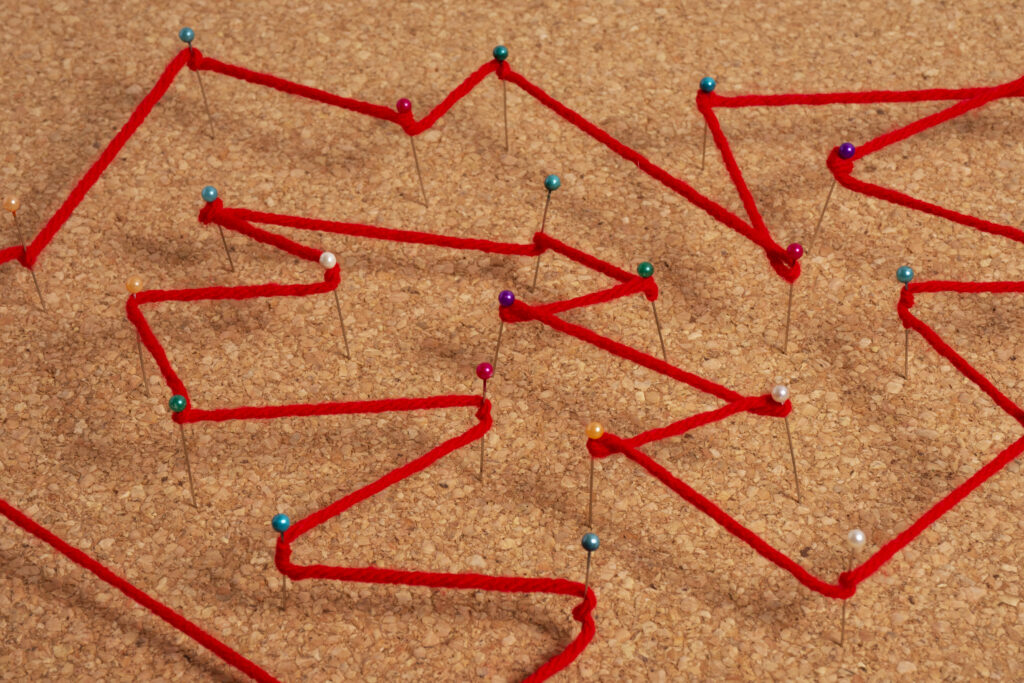
Data Collection
Foundation agents rely on large-scale interactive data collection to enhance their decision-making abilities. The process involves gathering vast amounts of diverse data from various sources, including physical environments and the internet. This data is crucial for training foundation agents to make informed decisions in real-world scenarios. Challenges such as data quality, bias, and privacy concerns must be addressed to ensure the effectiveness of data collection efforts. Opportunities lie in leveraging advanced technologies like machine learning algorithms to extract valuable insights from collected data.
Effective data collection is essential for improving the learning capabilities of foundation agents. By exposing these AI systems to a wide range of high-quality data sources, they can better understand complex patterns and relationships within different contexts. Diverse datasets enable foundation agents to generalize their decision-making processes across various situations, leading to more robust and reliable outcomes in practical applications.
- Foundation agents benefit from:
- Diverse datasets for comprehensive learning
- High-quality data sources for accurate decision-making
- Advanced technologies for efficient data processing
Pretraining Efficiency
Pre-training plays a vital role in equipping foundation agents with the necessary knowledge representations for decision-making tasks. By exposing these AI models to vast amounts of unlabeled data, they can learn underlying patterns and structures that are essential for making informed decisions. Efficient pre-training enables foundation agents to quickly adapt to new tasks by leveraging their existing knowledge base.
Efforts are being made to enhance the efficiency of pre-training processes for foundation agents. Strategies such as curriculum learning, self-supervised learning, and transfer learning are employed to optimize the utilization of unlabeled data during training. These techniques aim to improve the generalization capabilities of foundation agents while minimizing the need for extensive labeled datasets.
- Efficient pre-training leads to:
- Rapid adaptation to new tasks
- Enhanced generalization capabilities
- Optimization of unlabeled data utilization
Adaptation and Tuning
Adaptation and tuning are critical steps in optimizing the performance of foundation agents in real-world scenarios. Adapting these AI systems to new tasks and domains requires careful fine-tuning of their parameters and architectures. Challenges arise from domain shifts, where the characteristics of the target task differ significantly from those encountered during training.
Fine-tuning strategies play a key role in ensuring the adaptability and robustness of foundation agents. Techniques such as transfer learning, meta-learning, and reinforcement learning are utilized to facilitate quick adjustments based on new information or requirements. By continuously refining their models through adaptation and tuning processes, foundation agents can maintain optimal performance levels across diverse environments.
- Fine-tuning strategies include:
- Transfer learning for domain adaptation
- Meta-learning for rapid adjustments
- Reinforcement learning for continuous optimization
Knowledge Alignment
Aligning foundation agents with large language models is crucial for integrating world knowledge into their decision-making processes. This alignment enhances the ability of AI systems to leverage external information sources effectively when faced with complex problems or uncertainties. By incorporating world knowledge into their reasoning mechanisms, foundation agents can make more informed decisions based on a broader understanding of various domains.
Integrating world knowledge improves the decision-making capabilities and generalization skills of foundation agents. They can draw upon a vast repository of information spanning different fields and subjects, enabling them to tackle diverse challenges with greater accuracy and efficiency. World knowledge integration also enhances the problem-solving skills of foundation agents by providing them with valuable insights and context-specific information.
- Knowledge alignment benefits include:
- Improved decision-making capabilities
- Enhanced generalization skills
- Better problem-solving abilities
Deep Dive into Adaptation Methods
Fine-tuning strategies
Foundation agents can be customized for specific tasks through various fine-tuning strategies. By adjusting parameters and training data, agents adapt to new decision-making challenges. Fine-tuning enhances performance by tailoring the agent’s capabilities to unique requirements.
To improve accuracy, fine-tuning is crucial as it refines the agent’s responses based on real-world data. Fine-tuning ensures that the foundation agent can make informed decisions in complex situations. It allows for targeted adjustments that align with specific decision-making objectives.
In real-world applications, effective fine-tuning practices are essential for optimizing foundation agents. Best practices involve iterative adjustments, validation checks, and monitoring performance metrics. By following these methodologies, organizations can maximize the utility of their foundation agents.
Model adaptation
Adapting foundation agents to diverse scenarios presents both challenges and opportunities. The process of model adaptation involves updating algorithms and structures to accommodate new decision-making contexts. This flexibility enables agents to address a wide range of tasks effectively.
Continuous learning is key in enhancing the adaptability of foundation agents over time. Through model adaptation, agents evolve their decision-making capabilities based on feedback and experience. This iterative process ensures that the agent remains relevant and efficient in dynamic environments.
Optimizing performance requires a proactive approach towards model adaptation. Foundation agents must continually refine their strategies to meet evolving demands. By prioritizing adaptability and scalability, organizations can leverage foundation agents for sustained success.
Aligning Knowledge and Values
Ensuring ethical alignment
Foundation agents play a crucial role in shaping AI decision-making processes by aligning them with human values. To ensure ethical alignment, organizations can implement strategies such as regular ethical audits and reviews of the decision-making algorithms. These audits help identify any biases or deviations from ethical standards.
One of the significant challenges in embedding ethical considerations into AI decision-making is the inherent bias present in datasets used to train foundation agents. Biases in data can lead to discriminatory outcomes, highlighting the importance of continuously monitoring and adjusting algorithms to mitigate these biases effectively.
Transparency and accountability are key pillars in maintaining ethical alignment when deploying foundation agents. By providing clear explanations of how decisions are made and ensuring accountability for the outcomes, organizations can build trust with users and stakeholders, fostering a culture of ethical AI implementation.
Value-driven decision making
Value-driven decision-making processes facilitated by foundation agents prioritize incorporating human values and ethical principles into every decision. These agents are designed to not only optimize outcomes but also consider the broader societal impact of their decisions, promoting responsible AI applications.
Foundation agents leverage advanced algorithms to analyze vast amounts of data while simultaneously evaluating this information against predefined human values. This integration of values ensures that decisions align with ethical standards, contributing to more transparent and trustworthy AI systems.
Value alignment is at the core of promoting responsible and sustainable decision outcomes in AI applications. By prioritizing value-driven decision-making, organizations can uphold integrity, fairness, and inclusivity in their AI implementations, ultimately leading to positive societal impacts.
Multi-Model Integration
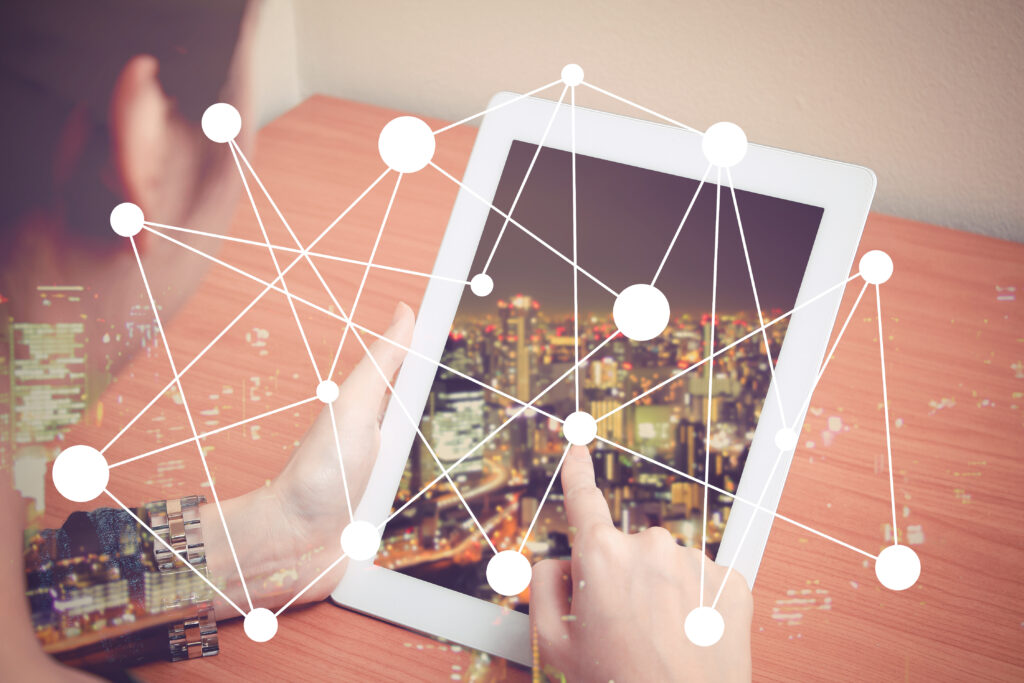
Effective Model Merging
Foundation agents leverage neural networks to enhance AI decision-making. Model merging is crucial for integrating different models effectively. By combining various neural network architectures, agents can achieve higher accuracy and robustness in decision-making tasks. The process involves merging multiple models into a unified framework, allowing them to complement each other’s strengths.
Challenges arise when merging models due to variations in architecture, training data, and optimization techniques. Despite these challenges, the benefits of model merging are substantial. It enables agents to leverage diverse perspectives from different models, resulting in more comprehensive decision-making capabilities. Model merging enhances the generalization ability of foundation agents by reducing overfitting and improving performance on unseen data.
Best practices for model merging include selecting models with complementary strengths, ensuring seamless integration through consistent data preprocessing, and fine-tuning the merged model to optimize performance. Regular evaluation and validation of the merged model are essential to maintain its effectiveness over time. By following these best practices, developers can create more robust and versatile AI systems that excel in real-world decision-making scenarios.
Enhancing Agent Capabilities
To enhance foundation agents’ capabilities, developers must explore advanced technologies such as reinforcement learning and meta-learning. These techniques enable agents to learn from experience and adapt their decision-making processes based on feedback received during interactions with the environment. By incorporating reinforcement learning, agents can improve their decision-making skills through trial-and-error learning.
Meta-learning plays a vital role in enhancing agent capabilities by enabling them to quickly adapt to new tasks and environments. This approach allows agents to generalize knowledge learned from previous experiences to solve novel problems efficiently. The continuous evolution of agent capabilities is essential to keep pace with the evolving demands of complex decision-making tasks in various domains.
Foundation agents undergo continuous improvement through iterative development cycles that focus on enhancing their learning algorithms, optimizing performance metrics, and expanding their knowledge base. By incorporating cutting-edge technologies and methodologies into agent development processes, developers can ensure that foundation agents remain at the forefront of AI decision-making capabilities.
Architectural Considerations
Deployment Strategies
Foundation agents play a crucial role in revolutionizing AI decision-making in real-world scenarios. Effective deployment strategies are essential for seamless integration. When deploying foundation agents, scalability, reliability, and performance optimization are key considerations.
To ensure successful deployment, it is important to consider scalability. Challenges related to scaling foundation agents in real-world applications can arise due to varying data volumes and complexities. Implementing strategies to enhance scalability is vital for accommodating growing datasets and evolving requirements.
In real-world applications, the flexibility of foundation agents is paramount. Designing flexible architectures allows foundation agents to adapt to diverse decision-making scenarios across different domains. Enhancing flexibility ensures that these agents can effectively address changing needs and environments.
Scalability and Flexibility
Scalability remains a critical aspect when deploying foundation agents into real-world applications. Enhancing scalability involves designing architectures that can handle increasing workloads and data volumes efficiently. Strategies like distributed computing can aid in improving scalability.
Flexibility is key for foundation agents to navigate the dynamic nature of real-world decision-making processes. By designing adaptable architectures, these agents can smoothly transition between different tasks and environments without compromising performance or accuracy.
In the realm of AI decision-making, the importance of flexibility cannot be overstated. Adaptable foundation agents can effectively respond to new challenges and requirements, ensuring optimal performance across various scenarios.
Overcoming Deployment Challenges
Real-world application hurdles
Implementing foundation agents in real-world applications often faces common hurdles and obstacles. Challenges related to data privacy, regulatory compliance, and user acceptance can hinder the seamless deployment of AI decision-making systems. Ensuring that sensitive data is protected while leveraging AI technologies is crucial.
Final Thoughts
You now grasp the potential foundation agents hold in reshaping AI decision-making. Embracing these innovations could propel your organization towards more informed, ethical, and efficient choices. By integrating diverse knowledge sources and aligning them with core values, you pave the way for robust decision frameworks that adapt and evolve with your needs. Overcoming deployment challenges is the final frontier to unleash the full power of foundation agents in your AI ecosystem.
Take action today. Start exploring how foundation agents can transform your decision-making landscape. Embrace the roadmap laid out, delve into adaptation methods, and navigate the integration complexities. Your journey towards enhanced AI decision-making begins now.
Related Article:
Featured Image courtesy of DALL-E by ChatGPT